Introduction
Understanding averages is a fundamental concept in data analysis. Averages provide a way to summarize and make sense of large sets of data by providing a single value that represents the central tendency of the data. In this article, we will explore the different types of averages – mean, median, and mode – and how to calculate them.
We will also discuss when to use each type of average and common mistakes to avoid when calculating averages. Additionally, we will explore real-world applications of average calculation in various fields such as finance, sports, and healthcare.
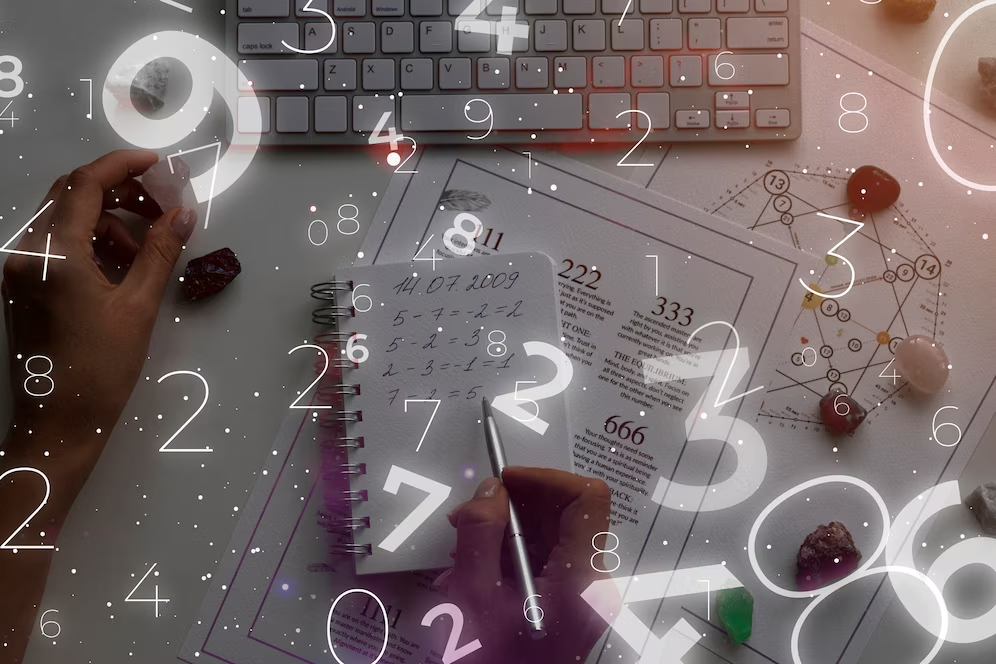
Understanding the Concept of Average
The average, also known as the arithmetic mean, is a measure of central tendency that represents the typical value in a dataset. It is calculated by summing up all the values in the dataset and dividing it by the total number of values. The average provides a way to summarize a large amount of data into a single value, making it easier to understand and compare different datasets.
Averages are widely used in data analysis because they provide a simple and intuitive way to understand the overall characteristics of a dataset. They can help identify trends, patterns, and outliers in the data. For example, in finance, the average return on investment can provide insights into the performance of a portfolio. In sports, the average score or time can help evaluate the performance of athletes or teams. In healthcare, the average age or weight can provide insights into the health status of a population.
Types of Averages: Mean, Median, and Mode
There are three main types of averages: mean, median, and mode. Each type of average has its own characteristics and is used in different situations.
The mean is the most commonly used type of average. It is calculated by summing up all the values in a dataset and dividing it by the total number of values. The mean is sensitive to extreme values or outliers in the dataset, which can skew the average. For example, if a dataset contains one extremely high value, the mean will be significantly higher than the other values in the dataset.
The median is the middle value in a dataset when it is arranged in ascending or descending order. If there is an even number of values, the median is calculated by taking the average of the two middle values. The median is less sensitive to extreme values compared to the mean, making it a better measure of central tendency for skewed datasets.
The mode is the value that appears most frequently in a dataset. It is useful for identifying the most common value or category in a dataset. Unlike the mean and median, the mode can be used for both numerical and categorical data.
How to Calculate Mean Average
To calculate the mean average, follow these steps:
1. Add up all the values in the dataset.
2. Count the total number of values in the dataset.
3. Divide the sum of the values by the total number of values.
For example, let’s calculate the mean average of the following dataset: 5, 7, 9, 11, 13.
1. Sum of values: 5 + 7 + 9 + 11 + 13 = 45
2. Total number of values: 5
3. Mean average: 45 / 5 = 9
Therefore, the mean average of the dataset is 9.
How to Calculate Median Average
To calculate the median average, follow these steps:
1. Arrange the values in the dataset in ascending or descending order.
2. If there is an odd number of values, the median is the middle value.
3. If there is an even number of values, calculate the average of the two middle values.
For example, let’s calculate the median average of the following dataset: 5, 7, 9, 11, 13.
1. Arrange the values in ascending order: 5, 7, 9, 11, 13.
2. There is an odd number of values, so the median is the middle value, which is 9.
Therefore, the median average of the dataset is 9.
How to Calculate Mode Average
To calculate the mode average, follow these steps:
1. Count the frequency of each value in the dataset.
2. Identify the value(s) with the highest frequency.
For example, let’s calculate the mode average of the following dataset: 5, 7, 9, 9, 11, 13.
1. Frequency of each value: 5 (1), 7 (1), 9 (2), 11 (1), 13 (1).
2. The value with the highest frequency is 9.
Therefore, the mode average of the dataset is 9.
When to Use Mean, Median, or Mode
The choice of which type of average to use depends on the characteristics of the dataset and the purpose of the analysis.
The mean is most appropriate when the dataset is normally distributed and does not contain extreme values or outliers. It provides a balanced representation of the data and is useful for comparing different datasets. However, if the dataset is skewed or contains extreme values, the mean may not accurately represent the central tendency of the data.
The median is more appropriate when the dataset is skewed or contains extreme values. It is less affected by outliers and provides a better measure of central tendency for such datasets. The median is also useful when the dataset contains ordinal or interval data that cannot be averaged.
The mode is most appropriate when the dataset contains categorical data or when identifying the most common value is important. It provides insights into the distribution of values in a dataset and can be used to identify trends or patterns.
Common Mistakes to Avoid When Calculating Average
When calculating averages, there are several common mistakes that should be avoided:
1. Forgetting to include all the values in the dataset: It is important to include all the values in the dataset when calculating the average. Leaving out values can lead to inaccurate results.
2. Using the wrong formula: Each type of average has its own formula, so it is important to use the correct formula when calculating the average. Using the wrong formula can lead to incorrect results.
3. Not considering the characteristics of the dataset: The choice of which type of average to use should be based on the characteristics of the dataset. Failing to consider the skewness or presence of outliers can lead to misleading results.
4. Rounding off the result too early: When calculating averages, it is important to carry out the calculations to sufficient decimal places before rounding off the result. Rounding off too early can lead to inaccurate results.
To avoid these mistakes, it is important to double-check the calculations and consider the characteristics of the dataset before calculating the average.
Real-World Applications of Average Calculation
Average calculation has numerous real-world applications in various fields. In finance, averages are used to analyze investment returns, evaluate portfolio performance, and calculate risk measures. For example, the average return on investment can provide insights into the profitability of an investment strategy, while the average volatility can help assess the risk associated with an investment.
In sports, averages are used to evaluate athlete or team performance. For example, the average score or time can help compare athletes or teams and identify trends or patterns. Averages are also used in sports analytics to analyze player performance and make strategic decisions.
In healthcare, averages are used to understand population characteristics and assess health outcomes. For example, the average age or weight can provide insights into the health status of a population. Averages are also used in clinical trials to analyze treatment outcomes and assess the effectiveness of interventions.
Accurate average calculation is crucial in these fields to ensure reliable analysis and decision-making. Mistakes in average calculation can lead to incorrect conclusions and potentially harmful outcomes. Therefore, it is important to use the appropriate type of average and avoid common mistakes when calculating averages.
Conclusion
Understanding averages is essential for data analysis in various fields. Averages provide a way to summarize large sets of data and make comparisons between different datasets. The three main types of averages – mean, median, and mode – each have their own characteristics and are used in different situations.
It is important to choose the appropriate type of average based on the characteristics of the dataset and the purpose of the analysis. Additionally, it is important to avoid common mistakes when calculating averages to ensure accurate results. Averages have real-world applications in finance, sports, healthcare, and other fields, where accurate average calculation is crucial for reliable analysis and decision-making.